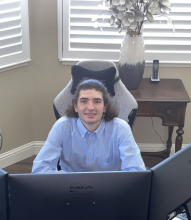
Major:
University:
Mentor(s):
Faculty Sponsor(s):
Faculty Sponsor's Department(s):
Project Title:
Project Description:
Non-destructive evaluation techniques are popular for characterizing and monitoring microstructural damage accumulation in real-time. Acoustic emission (AE) is one such technique that uses piezoelectric transducers to monitor the strain waves that are emitted when cracks propagate in a bulk material. In materials systems hosting multiple damage mechanisms, one promising application of AE is to map an AE signal to the damage mechanism which emitted it. However, the damage state in a material under load continuously evolves and tracking changes to waveform features under these conditions is difficult. Therefore, computational techniques are needed to help assign a waveform to the damage mechanism that emitted it. Machine learning (ML) is one computational method that is well suited to addressing this problem. However, there is currently no unified ML framework for damage mechanism identification. This prevents the broader implementation of such frameworks in scientific investigations and engineering applications. It is therefore necessary to compare efficacies of existing AE-ML frameworks.
To this end, two sets of transducers are used to collect waveforms that are generated from monotonic tension tests. Existing ML frameworks from literature are applied to cluster the waveforms. The clusters determined by the individual frameworks were then assigned to damage mechanisms using domain knowledge. The efficacy of a single framework was assessed by comparing the consistency of clusters between different sensors using the adjusted Rand index. Based on this metric, the AE-ML framework that produced the most precise results involved a two-step approach where data was first fit with a self-organizing map which was subsequently clustered with the k-means algorithm. It was found that although this framework was precise, it was not accurate and therefore should not be used in future AE-ML studies.